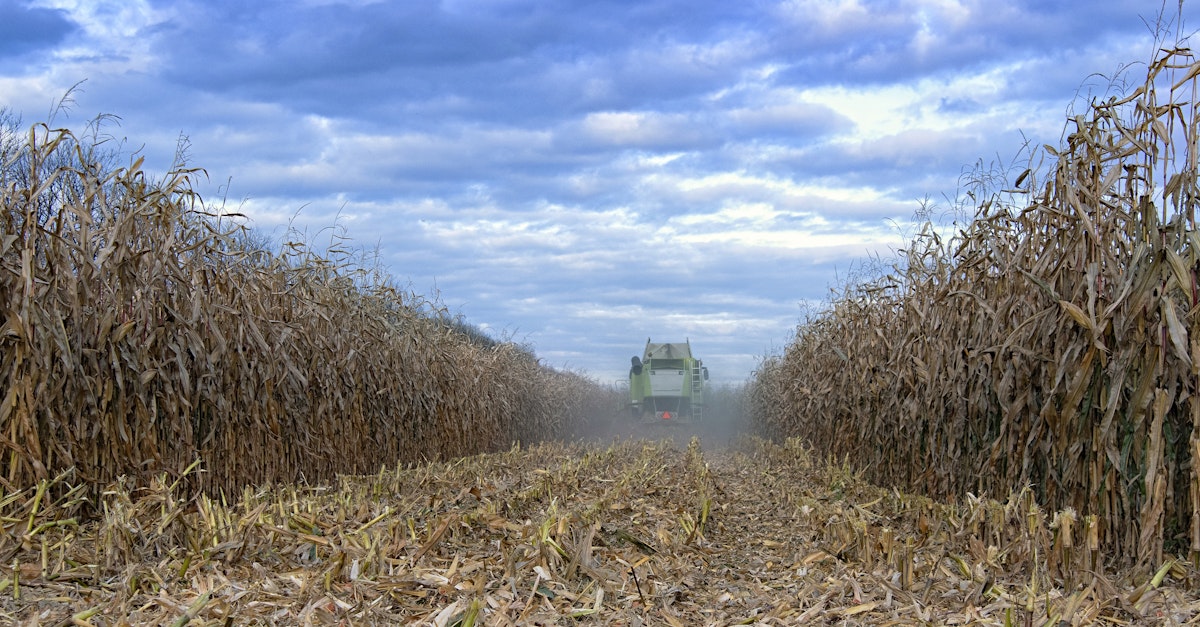
Steep learning curve
I’m sure you’ve all heard of the term ‘a steep learning curve’ and, well… after my first two months as part of the team at Leaf, I can honestly say that I know exactly what this means. Having been in Agtech for over 4 years already I thought I knew a lot about data in agriculture, but it turns out I still have a lot to learn. One of the main principles that I’ve learned at Leaf so far is that ‘it’s important to keep it clean’. Seeing as I’m not just talking about cleaning your truck or boots, I thought it valuable to put some of my learnings on paper.
A good starting point in this discussion is Rhishi Pethe’s recent newsletter, in which he elaborates on the hub and spoke model and why it is just as relevant to data in agriculture now, as it was to the airline industry in the 1950s. Rhishi explains that if 9 companies want to connect point to point, 36 connections have to be created, whereas only 8 connections are needed in a hub and spoke model.
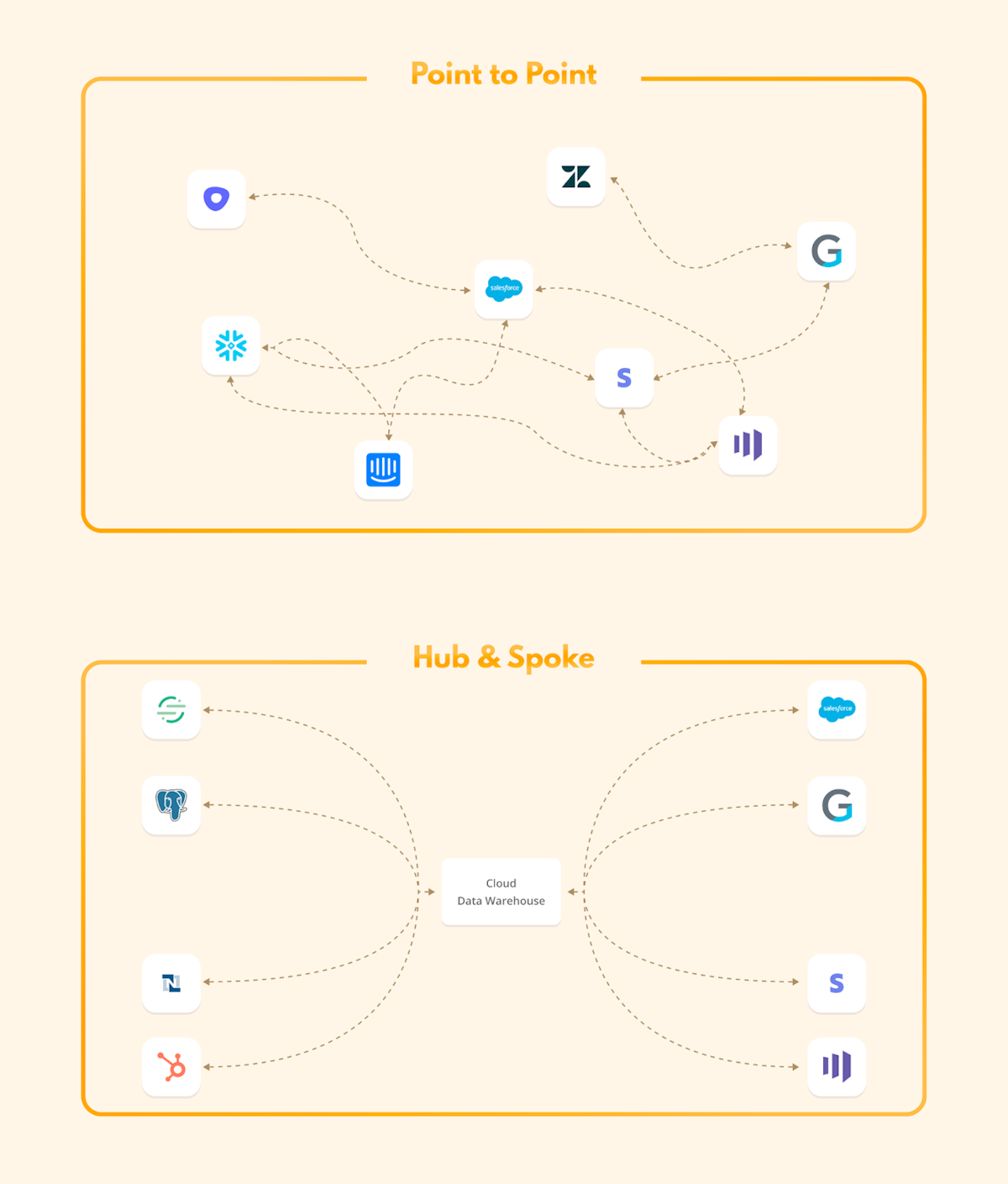
Image from Hub-and-Spoke vs. Point-to-Point Data Synchronization: There's One Clear Winner
"This ‘clean’ way of connecting an industry is key to reducing friction in interoperability..."
This ‘clean’ way of connecting an industry is key to reducing friction in interoperability, next to the fact that “many organizations (especially retailers and cooperatives) might not have the engineering resources to do many multiple point to point integration to create additional value for their customers or members,” according to Rhishi. In other organizations with large development teams, this “clean” way of handling interoperability means that those engineers can focus all of their effort on their core products.
A second important ‘clean’ aspect about ag data relates to the way data is structured. Every OEM in agriculture provides data in a different way, through novel file formats, different decimal precision, different coordinate systems, different units, different naming structures, different frequencies of data collection, etc. Those Agtech businesses that ingest data directly from OEMs have to ensure that all this data gets converted into a consistent format so that it can be used in downstream applications. Not a cheap, easy, or quick feat to achieve for anyone, and a major distraction for most that try.
The third ‘clean’ feature is the content of the data. Just because data gets collected by a piece of machinery and is brought into a consistent format and structure doesn’t mean that it is clean, correct and useful. Take yield data as an example: through a variety of equipment related factors (unexpected stop in the middle of a field, feeder house blockage, etc.), all yield data contains values that are statistical outliers, which in almost every case should be filtered out in order to not distort the data set for a field as a whole.
For example: an ag retailer that wants to provide yield data analysis services to their grower clients, but they run a mix of combines and equipment (John Deere, Case IH combines, and other equipment retrofitted to map their harvest with FieldView). By working with Leaf, these ag retailers can receive data from all different brands of yield monitors through the same API endpoint. Plus, when they receive the data, it is already all in a consistent, aggregated and standardized JSON file format.
Because the yield data has passed through the Leaf ‘hub’, it has also been cleaned, which means that the data has been filtered to remove all points with values that are seen as outliers. For those wanting to see the full details of how this works, and how the configurations of this filter can be customized, you’ll want to check out this link. For those interested in the non-technical and visually pleasing results, see the example of unfiltered vs filtered yield data below:
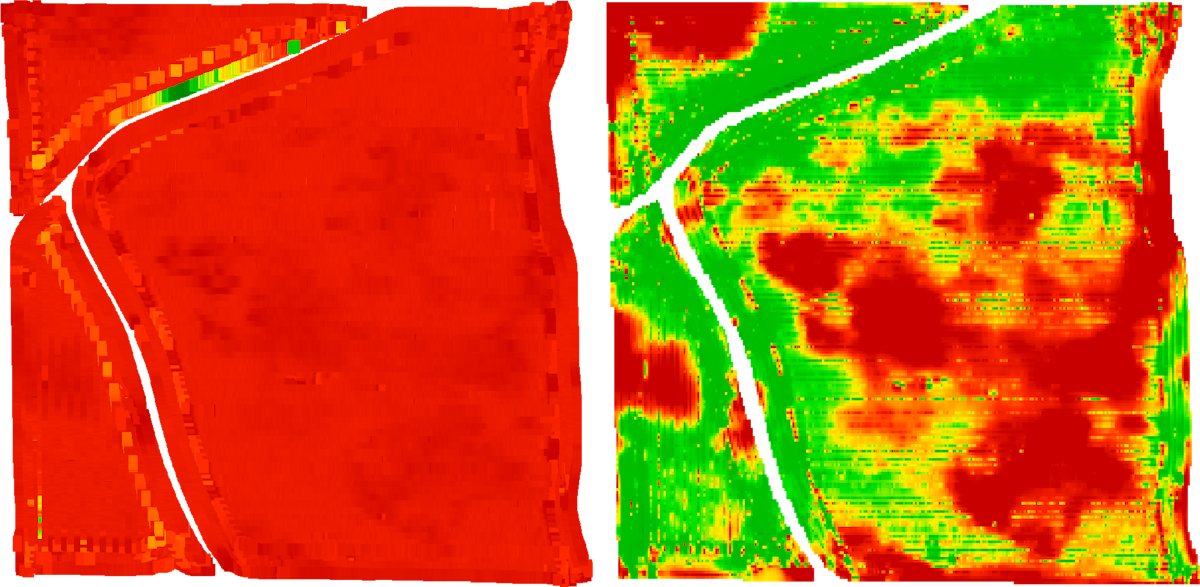
Images from Leaf: Previous (unfiltered) vs. New (filtered)
Data filtering or processing is not part of most OEM’s standard offering but, as you can see, using unfiltered data can be ‘dangerous’ and offer an incorrect interpretation of what actually occurred in the field.
So yes, ‘keeping it clean’ is important in ag data, and harvest data is just one of many examples I could have used. If you are interested to learn more about letting your data flow through Leaf, or if you’re just after some solid cleaning tips, I look forward to hearing from you!
Get a demo